Exploring Key Concepts in Statistics Education
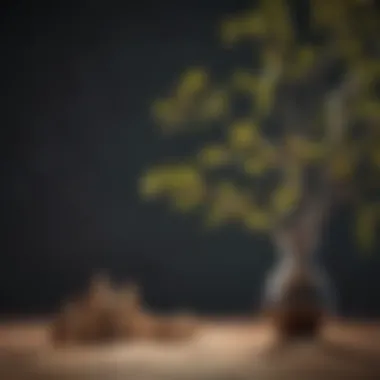
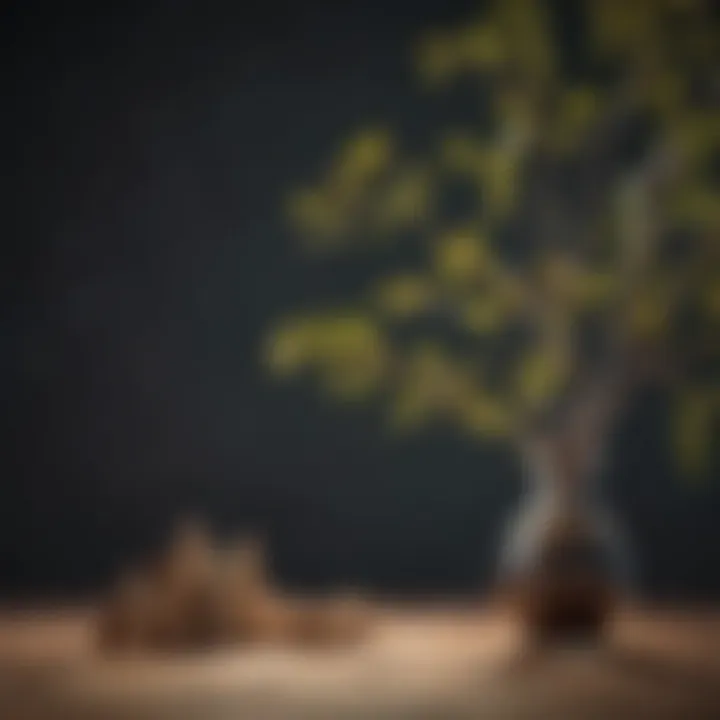
Intro
Statistics is a field that often feels like a minefield to the uninitiated. Many people either steer clear of it or regard it as a dull topic reserved for the mathematically inclined. However, grappling with statistics can actually be a cornerstone in making informed decisions, more so in the realm of finance. The core curriculum of statistics is not just a bunch of numbers and formulas; it encompasses vital soft skills and practical knowledge that can empower you in today's data-driven world.
In the following sections, we will explore foundational concepts and techniques encountered within statistics classes. By breaking it down, we aim to show not just how these concepts are learned, but their broader relevance, especially to newbie investors and seasoned pros alike. Through understanding this core curriculum, readers can elevate their financial literacy and approach investment decisions with a sharper edge.
Key Financial Concepts
Before sailing into the nuances of data analysis, itās essential to get our ducks in a row. The first step is understanding the key terms and principles that anchor the world of finance and statistics.
Definitions of Essential Terms
In any statistical endeavor, definitions lay the groundwork. Here are some crucial terms you will often come across:
- Mean: This is essentially the average. When you sum all values in a dataset and divide by the count, you get the mean.
- Median: This is the middle value in a dataset when the numbers are arranged in order. If thereās an even number, you take the average of the two middle numbers.
- Mode: The mode refers to the number that appears most frequently in your dataset.
- Standard Deviation: A measure that tells you how spread out the numbers are in your data set. A low standard deviation suggests that the data points tend to be close to the mean.
- Correlation: This statistic measures how two variables move in relation to each other. A positive correlation means they move in the same direction, while a negative correlation indicates the opposite.
Understanding these terms is like having a toolbox. Each tool serves a different purpose, and knowing how to use them is fundamental in any data-driven decision-making.
Explanation of Key Financial Axioms
Now that youāre somewhat familiar with terminology, let's get into some core axioms that guide financial decision-making:
- The time value of money: This principle posits that money today is worth more than the same sum in the future due to its potential earning capacity. This concept is the foundation for many investment decisions.
- Risk and return relationship: Higher return investments generally come with higher risk. Itās crucial to assess how much risk youāre willing to take for the expected return.
- Diversification: Spreading your investments across various asset classes reduces the impact of any one poor-performing investment on your overall portfolio.
- Market efficiency: This axiom suggests that stock prices reflect all available information, making it challenging to consistently achieve higher returns than the market average.
Practical Skills for Applying these Concepts
In the classroom, aspiring investors aren't just plugged into theory. Tools such as Excel or statistical software have become essential for applying these core concepts in real-life scenarios. Being adept with these tools allows one to manipulate data sets, conduct analyses, and visualize trends effectively.
These foundational ideas prepare you for more complex topics down the line, helping you to confidently navigate your financial landscape. Whether you're just dipping your toes into statistics or looking to refine your skills, grasping these concepts will set a robust groundwork for deeper understanding.
Prolusion to Statistics Education
Statistics education plays a critical role in shaping the way individuals approach decision-making, especially in environments saturated with data. Understanding statistics provides a foundation for critically evaluating information, making informed decisions, and developing analytical skills. In todayās world, where misinformation can spread like wildfire, possessing statistical literacy is no longer a luxury; itās a necessity.
The Importance of Statistical Literacy
Statistical literacy is akin to being fluent in a language. It equips individuals with the ability to interpret data, understand trends, and analyze results rather than merely accepting figures at face value. This fluency goes beyond just numbers; it fosters a mindset that challenges assumptions and encourages questions. For instance, when faced with data related to investment performance, savvy investors can separate genuine insights from mere hype, allowing them to make sound financial choices.
Moreover, statistical literacy impacts various life domains, empowering individuals to:
- Navigate Health Decisions: Understanding clinical trial data can affect choices about treatments or health insurance plans.
- Engage in Civic Activities: Being able to interpret statistical data from surveys enhances participation in democratic processes, such as voting.
- Enhance Career Opportunities: Many professions, from marketing to engineering, rely heavily on data analysis and interpretation.
Essentially, statistical literacy underpins the ability to translate numbers into manageable insights that drive personal and professional growth.
Overview of the Statistics Curriculum
The curriculum for statistics education is designed to progress from the foundational concepts to more complex analytical methods. It aims to build not only knowledge but the necessary skills to handle real-world data.
Typically, a well-rounded statistics program includes:
- Fundamentals of Data Types: Students learn about qualitative and quantitative data, which sets the groundwork for deeper analysis.
- Descriptive Statistics: Instruction on measures of central tendency, like mean and median, as well as measures of dispersion, like range and standard deviation.
- Inferential Statistics: Exploring sampling methods and hypothesis testing illustrates how we can make assumptions about a larger population based on a small sample.
- Data Visualization: A strong emphasis on graphical representations further aids in understanding and interpreting data.
- Practical Application: Ultimately, tying theoretical concepts back to practical uses in fields such as finance, market research, and policy-making reinforces students' comprehension and application abilities.
In summary, the curriculum is much more than a series of lessons; it's a pathway that guides students through the maze of data interpretation and application, preparing them not only academically but also for the complexities of modern life.
Statistical literacy not only helps in understanding data but also builds a more informed society where decisions are based on analysis rather than assumption.
Fundamental Concepts in Statistics
The world of statistics is richly layered, offering a realm where numbers take form to convey realities. Fundamental concepts in statistics provide the scaffolding upon which more complex ideas are built. Grasping these core principles is akin to having a roadmap for navigating various statistical methods and applications. This section delves into two critical realms: understanding data types and the role of descriptive statistics. By paying attention to these facets, readers can develop a strong foundation, enabling them to tackle practical applications in various fields, especially in investing.
Understanding Data Types
Understanding data types is crucial because it affects how we analyze information. Knowing whether data is qualitative or quantitative can shape the methodologies applied in research or financial analysis.
Qualitative vs. Quantitative Data
Qualitative data refers to non-numerical information that describes categories or characteristics. This type of data can include things like opinion polls or survey responses where the choices may be labels such as "satisfied," "neutral," or "dissatisfied." The key characteristic here is that qualitative data helps capture sentiments and perspectives, which are vital for stakeholder engagement. On the flip side, quantitative data involves numerical measurements and signifies quantities or amounts. For instance, figures such as sales revenue or market share fall under this category.
The unique feature separating these two is their representational nature. For instance, if a financial analyst wants to interpret customer feelings towards a product launch, qualitative data may provide depth, while quantitative data can reveal trends based on numerical performance. One advantage of qualitative data comes from its rich detail; it can tell a story behind the numbers that quantitative data often can't capture alone. However, qualitative data can be subjective, whereas quantitative data provides more objective analysis but might miss the deeper human elements.
Discrete vs. Continuous Variables
When diving into the types of quantitative data, distinguishing between discrete and continuous variables becomes fundamental. Discrete variables can take on a finite number of values, often counting items such as the number of employees in a company or cars sold within a month. Continuous variables, on the other hand, can take on an infinite number of values within a given range, such as temperature or height.
This distinction is crucial in choosing the right statistical methods for data analysis. Discrete variables lend themselves well to methods like chi-square tests, while continuous variables can be analyzed through regression models. The unique aspect of discrete variables lies in their ability to represent whole units, making them easy to identify and quantify in contexts like market segmentation. Continuous variables, while offering more depth in measurements, can complicate analyses due to their fluid nature and potential for variability.
The Role of Descriptive Statistics
Descriptive statistics play a vital role in summarizing data sets, providing a clearer picture without diving into complex analysis. These statistics convert data into digestible insights, which facilitates decision-making.
Measures of Central Tendency
Measures of central tendencyāmean, median, and modeāserve as essential tools for understanding the crux of a data set. The mean is the average value, the median represents the middle value when data is ordered, and the mode indicates the most frequently occurring number. These metrics can provide a snapshot of the data's overall behavior.
The significance of these measures is in their capacity to give a quick overview of large volumes of data. For a financial analyst trying to gauge typical investment returns, leveraging the mean can provide clarity amidst the noise. However, while useful, these measures can sometimes conceal underlying variations in data, especially if the data set contains outliers.
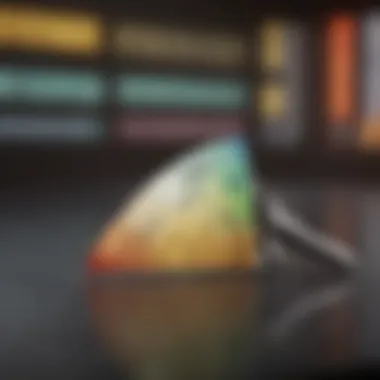
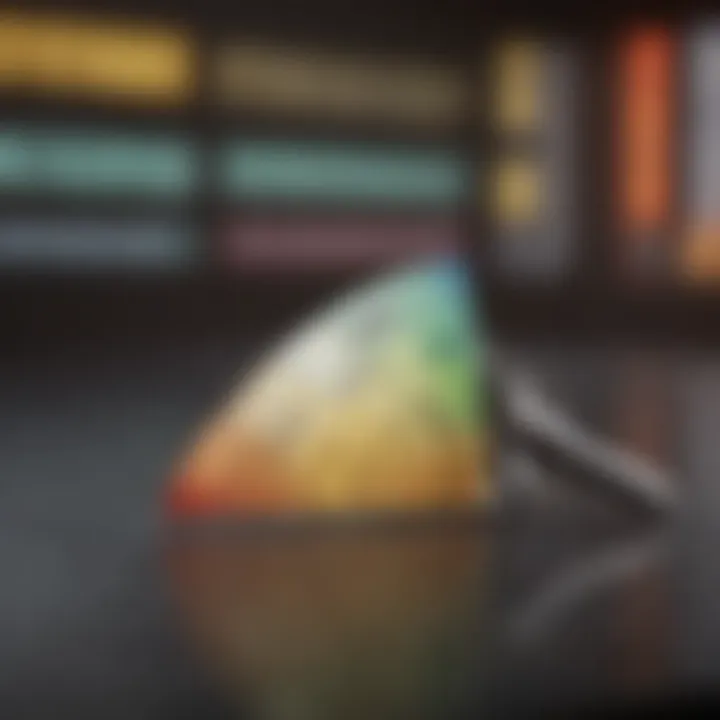
Measures of Dispersion
Moving beyond central tendency, measures of dispersionāsuch as range, variance, and standard deviationāare essential for understanding the spread of data. For example, range indicates the difference between the highest and lowest values, while variance and standard deviation illustrate how much data points deviate from the mean. Understanding this variation is particularly useful in finance, as it helps assess risk and volatility.
By analyzing measures of dispersion, investors can make better-informed decisions. For instance, if two investments have the same average return, but one shows a higher standard deviation, it indicates greater risk. Thus, having a grasp of dispersion metrics is indispensable for effective financial planning and personal risk management.
"Descriptive statistics don't just summarize data; they breathe life into numbers, allowing for thoughtful interpretations and informed strategies."
This overview of fundamental concepts in statistics lays a sturdy groundwork for delving into more advanced methods, ensuring that readers not only understand the 'how' but also the 'why' behind statistical analysis.
Inferential Statistics Explained
Inferential statistics serves as a critical component of statistical education, bridging the gap between raw data and meaningful conclusions that can guide decision making. In simple terms, it allows us to make predictions or inferences about a population based on a sample of data. This section unpacks why understanding inferential statistics is pivotal for both academic pursuits and practical applicationsāespecially for those delving into the intricacies of financial analysis.
Sampling Techniques and Their Importance
When it comes to inferential statistics, sampling is the foundation upon which sound conclusions are built. Proper sampling techniques ensure that the data collected is representative of the broader population, allowing for generalizations that hold water.
Random Sampling
A defined aspect of random sampling is its ability to give each member of a population an equal chance of being selected. This key characteristic bolsters the reliability of the data and minimizes bias. For readers looking into statistical methodologies, this method is often favored because it simplifies the generalization process and strengthens the validity of conclusions drawn from the sample.
The unique feature of random sampling lies in its simplicity. By using tools such as random number generators or lottery-style methods, researchers can easily select samples without pulling their hair out over selection bias. However, it is worth noting that while it has its perks, random sampling also has drawbacks, such as potential variability in results across different samples. Hence, itās essential to recognize its benefits alongside its limitations in a focused discussion of statistical concepts.
Stratified Sampling
In contrast, stratified sampling divides the population into distinct subgroups, or strata, and samples proportionately from each. This method shines in its capability to reflect the characteristics of the population accurately, especially when working with diverse groups. For this article, stratified sampling is particularly beneficial, as it facilitates targeted analysis, which is vital in financial and market research segments.
The unique feature of stratified sampling is its precision in ensuring all subgroups are represented in the analysis. This means that when outcomes are drawn, they arenāt skewed by over-representation of one section. However, this method can be complex and time-consuming, calling for careful planning to define strata correctly.
Making Inferences from Sample Data
Inference is where the rubber meets the road in statistics. Making educated guesses about populations from sample data is an art and a science, and it hinges on techniques like confidence intervals and hypothesis testing.
Confidence Intervals
Confidence intervals provide a range of values that is likely to contain the population parameter. A defining aspect of confidence intervals is their role in quantifying uncertainty around a sample estimate. For anyone operating in the realms of statistics and finance, this makes confidence intervals an invaluable tool as they offer a safety net in interpretations.
The benefit of confidence intervals lies in their communicative power; they not only present a point estimate but also frame the uncertainty surrounding that estimate. However, one could argue that confidence intervals are susceptible to misinterpretation. It's key to understand that a wider interval might indicate greater uncertainty and can be a hindrance when trying to make decisive conclusions.
Hypothesis Testing
Next up is hypothesis testing, a method that evaluates assumptions (hypotheses) about a population using sample data. This statistical technique is characterized by its structured approachāformulating a null hypothesis and an alternative hypothesis allows a clearer pathway for analysis. Itās commonly utilized in various sectors, helping to validate or reject assumptions based on empirical evidence.
The unique feature of hypothesis testing is its binary nature; it's either supporting the null hypothesis or providing grounds to accept the alternative. However, such a rigorous structure can also lead to misconceptions. Many folks misunderstand that failing to reject the null hypothesis does not prove it true, just that there's insufficient evidence against it. Thus, while hypothesis testing is powerful, it's equally important to grasp its potential pitfalls in data interpretation.
Data Visualization Techniques
Data visualization is a crucial element in the statistics curriculum, offering a visual representation of data that can make complex information easier to digest. In a world awash with numbers, turning those figures into meaningful graphics is like transforming a jigsaw puzzle into a clearer image. Not only does it enhance understanding, but it also serves as a powerful tool for communication. When data is presented visually, it can reveal patterns, trends, and correlations that might otherwise remain hidden in raw datasets.
The effectiveness of visualization lays in its ability to distill information. An investor glancing at a chart can often glean essential insights within seconds, far quicker than if they poured over spreadsheets full of numerical data. Essentially, visualization allows people to see the proverbial forest for the trees.
The Importance of Graphical Representations
Graphical representations serve as a bridge between data and comprehension. They allow observers to quickly grasp relationships between variables, identify outliers, and recognize any trends over time. For instance, when looking at financial data, a line graph might show a steady increase in stock prices, while a pie chart could illustrate how much share one company holds in relation to its competitors.
By using these visuals, educators and analysts can enhance learning outcomes and facilitate more effective decision-making. Itās much easier to convince a skeptical audience of a point when you can show them the essence of your argument in a straightforward graphic. These representations not only foster engagement but can also shape policy or investment strategies, making them indispensable in the field of statistics.
Common Types of Charts and Graphs
Bar Charts
Bar charts are a staple in the visual toolkit. They display data with rectangular bars, where the height or length of the bar is proportional to the value it represents. This format lends itself easily to comparisons.
A standout feature of bar charts is their clarity in comparing multiple categories. If investors are trying to gauge the performance of different stocks within a sector, a bar chart can succinctly highlight which stocks are outpacing others. The straightforward nature of bar charts makes them a go-to choice in the classroom as well as the boardroom. However, one must be cautious: overcrowding the chart with too many bars can lead to confusion rather than clarity.
Scatter Plots
Scatter plots take a different approach by showing the relationship between two or more variables. Each point on the graph represents an observation, allowing for quick visual analysis of correlations. For example, one could depict annual income against spending on entertainment, measuring how these variables interact.
The unique feature of scatter plots is their ability to reveal the direction and strength of relationships. This can help investors make educated assessments on whether higher spending can be associated with growing income, for example. While scatter plots are informative, they can become less practical if there are too many overlapping points, obscuring meaningful patterns.
Histograms
Histograms are another key visualization technique, focusing primarily on frequency. Instead of discrete categories, they divide data into ranges, making it possible to observe the distribution of values within those ranges. For instance, a histogram can effectively show how many investments fell into various return brackets over a given timeframe.
A major attribute of histograms is their capacity to illustrate distribution shapes, such as normal or skewed distributions. This can be particularly enlightening for investors and analysts who need to assess risk and consider the broader context behind the numbers. However, their reliance on binning can sometimes obscure finer details in the data, particularly if bars are not set at an ideal width.
In summary, employing these visualization techniques not only enhances understanding but also equips investors and analysts with the necessary tools to interpret and communicate data effectively. The proper graphical representations can transform ordinary statistics into impactful insights, making data a powerful ally in decision-making across various domains.
"Visualization isnāt just presentation; it is understanding. It transforms raw numbers into compelling narratives that can shape strategies and foster informed choices."
By harnessing these visualization techniques, both novice and seasoned investors can navigate the complexities of data with confidence, making it a fundamental aspect of modern financial decision-making.
Statistical Software and Tools
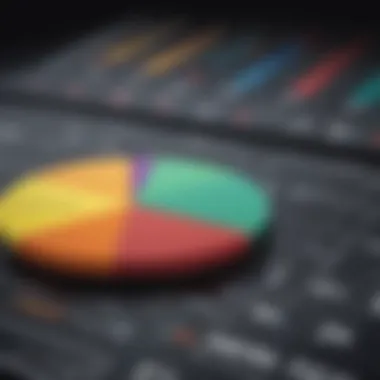
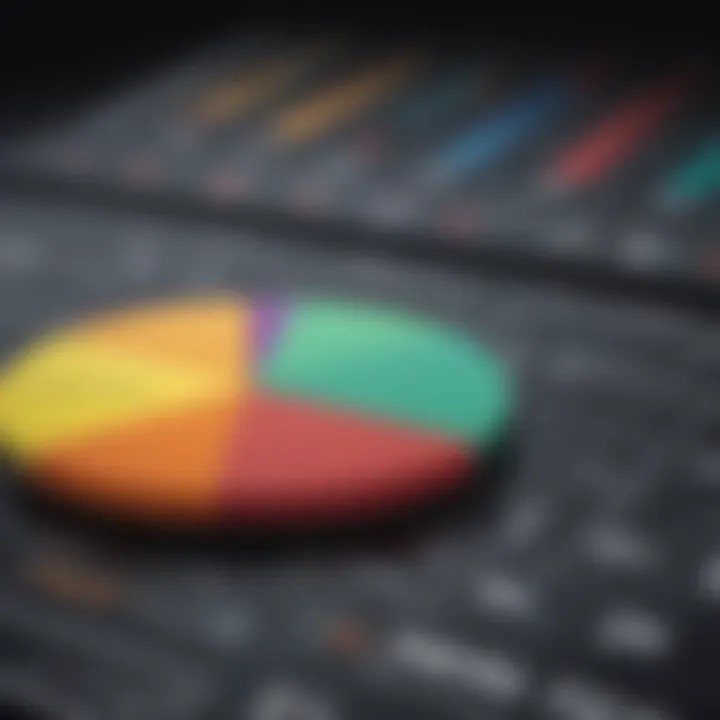
When diving into the world of statistics, one quickly realizes the importance of statistical software and tools. They act as a backbone for processing data, running analyses, and visualizing results. Whether you are a beginner or a seasoned investor, the ability to manipulate and interpret data efficiently can set you apart. With numerous options available today, understanding which software to choose and how to effectively use it is essential for solidifying your statistical literacy.
Preface to Statistical Software
Statistical software programs have revolutionized the way we analyze data. No longer do you need to crunch numbers by hand or rely solely on calculators. Software applications not only save time but also enhance the accuracy of computations. They come equipped with numerous features that allow users to perform complex analyses easily. From automating repetitive tasks to providing advanced reporting tools, the role of statistical software in education and practical applications cannot be understated.
Equipped with user-friendly interfaces, these programs cater to both novices and experts alike, making statistics more accessible. Importantly, they help bridge the gap between theoretical knowledge and practical skills, enabling users to engage deeply with statistical concepts.
Software for Data Analysis
Data analysis can be a daunting task without the right tools. Different software options exist, each bringing its own unique strengths and capabilities, catering to various user needs. Here, weāll explore two prominent choices for data analysis: Excel and programming languages like R and Python.
Excel for Statistics
Excel is often the first software that comes to mind for many individuals venturing into data analysis. This popularity stems from its user-friendly interface and widespread availability. Basic statistical functions are built right into Excel, allowing users to calculate averages, standard deviations, and correlation coefficients with ease.
One unique feature of Excel is its PivotTables, which enables users to summarize data quickly and draw insights without getting lost in the details. However, while Excel is an effective tool for imitated or straightforward data tasks, it has limitations. The handling of large datasets can slow down performance, and advanced statistical techniques often require more sophisticated tools.
Thus, while Excel serves as an excellent entry point for beginners, users seeking more depth may eventually outgrow its capabilities.
R and Python Overview
When we look past Excel, two titans emerge: R and Python. Both are robust programming languages equipped for statistical analysis, and they offer extensive libraries designed for various tasks in data science.
R is particularly favored in academic circles for its strength in data visualization and statistical testing. Its built-in graphing capabilities let users create stunning visual representations of data with relative ease. Meanwhile, Python has carved out its niche in the finance and technology sectors. It is revered for its versatility and extensive community support. The Pandas library, for instance, is a powerful tool for data manipulation, while Matplotlib facilitates the creation of complex plots.
Both R and Python require a learning curve, making them less appealing for complete beginners. But their adaptability and functionality ultimately provide a rewarding experience for those willing to invest the time. The choice between R and Python largely depends on the specific needs of the user, whether it be complex analytics or integration with web applications.
"Statistical software is not just a tool; it is an extension of the mind, enabling analysts to turn data into actionable insights."
Practical Applications of Statistics
The practical applications of statistics cannot be overstated, especially in a world that increasingly relies on data-driven decisions. Regardless of one's field, understanding how to analyze and interpret data can be a game changer. By acquiring statistical skills, individuals can make informed choices based on solid empirical evidence rather than gut feelings or mere assumptions. In particularly fast-moving sectors, such as finance or marketing, statistical acumen is necessary to remain competitive.
Statistics in Financial Analysis
When it comes to financial analysis, statistics serve as the backbone for risk assessment and investment strategies. A key aspect here is the ability to interpret relevant data trends and patterns. For instance, investors often turn to historical performance data to evaluate the potential returns on various investments. Simple metrics like the average return can provide a baseline, but digging deeper into standard deviations or variance helps assess the risk involved. This is crucial; as one industry saying goes, "Higher returns often come with higher risks."
Moreover, tools such as regression analysis are vital. This technique allows analysts to understand how different variablesālike interest rates or economic growthāaffect stock prices. More sophisticated models may leverage multiple regression to untangle complex relationships between numerous factors, helping investors not miss a beat in volatile markets.
Using Statistics in Market Research
Market research is another significant area where statistical concepts come into play. Businesses and entrepreneurs canāt afford to guess what consumers want. Here, statistics helps with crafting effective marketing strategies. After conducting surveys, for example, businesses utilize statistical analysis to interpret consumer preferences and behaviors. This data can shed light on market size, potential customer segments, and even predict future trends.
In terms of practical benefits, using statistics to inform marketing decisions leads to increased efficiency. Companies donāt just throw darts at a board; they launch targeted campaigns that resonate with specific demographics, guided by data insights. This means that fewer resources are wasted on ineffective advertising efforts.
"Statistics is the science of learning from data."
Ultimately, in both financial analysis and market research, the ability to wield statistics translates into smarter, more strategic decisions. Mastering these tools can be the difference between success and stumbling blindly.
Advanced Statistical Techniques
In the realm of statistics, advanced techniques form the backbone of rigorous analysis and data interpretation. As financial landscapes evolve, the need for sophisticated methods becomes imperative. This section delves into two fundamental aspects of advanced statistical techniques: regression analysis and statistical decision making. Understanding these topics equips both beginners and seasoned investors with the analytical toolkit necessary to make informed decisions, ultimately enhancing their ability to interpret data in a meaningful way.
Regression Analysis
Regression analysis is a powerful tool that allows us to explore relationships between variables. By examining how the dependent variable responds to one or more independent variables, we can unveil patterns that drive outcomes. This analysis is particularly advantageous in financial scenarios, helping investors understand trends and predict future movements.
Simple Linear Regression
Simple linear regression focuses on the relationship between two variables, where one assumes a linear relationship. Itās like drawing a straight line through a scatter of points, serving as a guide. One pivotal feature of this method is its simplicity; it enables quick insights without the convoluted mathematics often associated with more intricate models.
- Benefits of Simple Linear Regression:
- Unique Features:
- Quick and straightforward computation.
- Great for establishing basic trends.
- Strong interpretability:
- By analyzing the slope, one can directly interpret the impact of the independent variable on the dependent one.
- Assumes a constant rate of change between the variables.
- Works well with small data sets and linear relationships.
While simple linear regression shines in many areas, it does have its limitations. It can fall short when the relationship isnāt truly linear or when dealing with more than two variables. Thus, for a comprehensive understanding, it lays the groundwork but often spurs the need for more advanced methods.
Multiple Regression
Multiple regression expands upon the concept of simple linear regression by analyzing the impact of multiple independent variables on a single dependent variable. Imagine trying to make sense of a recipe where several ingredients influence the final dish ā thatās what multiple regression does with data. This technique allows a more nuanced understanding of the forces at play.
- Benefits of Multiple Regression:
- Unique Features:
- Ability to consider the effects of various factors simultaneously.
- Provides a more comprehensive model of reality, which is vital in fields like finance.
- Helps in identifying significant predictors amid various inputs.
- Can handle collinearity among independent variables, but requires caution.
- More complex computations and interpretations compared to simple linear regression.
Though powerful, multiple regression can lead to overfitting if too many variables are included. Careful consideration and experience are essential in balancing the modelās accuracy with interpretability.
Statistical Decision Making
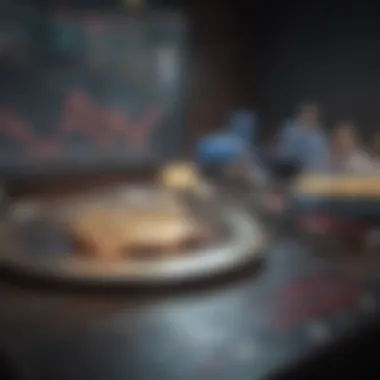
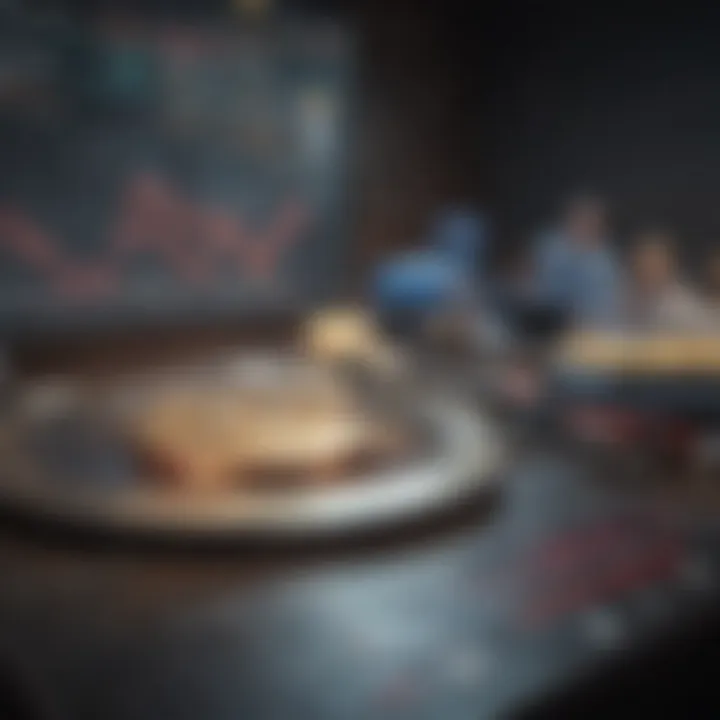
Statistical decision making revolves around analyzing and interpreting data to support informed choices. At the heart of this process lie the concepts of p-values and statistical significance, which play crucial roles in testing hypotheses and drawing conclusions from data.
Understanding p-values
P-values offer a statistical measure that helps determine whether an observed effect is due to chance or represents a genuine effect. When conducting hypothesis tests, p-values inform us about the probability of observing results as extreme as those gathered under the null hypothesis. A lower p-value suggests stronger evidence against the null hypothesis.
- Key Characteristics:
- Benefits:
- Unique Features:
- Provides a quantifiable measure to support or reject a hypothesis.
- Common threshold is often set at 0.05.
- Helps assess the reliability of the results.
- Offers a straightforward framework for hypothesis testing.
- Does not indicate the size of an effect or its importance.
- Misinterpretation can lead to erroneous conclusions.
Understanding p-values is an essential skill, but must be used alongside other statistical context to draw forthright conclusions.
Statistical Significance
Statistical significance tells us whether findings from our data can be considered meaningful in the broader context. It goes beyond mere chance and highlights reliable results that warrant further investigation. A key element of statistical significance is its connection to hypothesis testing, demonstrating how strongly evidence conflicts with the null hypothesis.
- Benefits:
- Key Characteristics:
- Unique Features:
- Clarifies which results are noteworthy and worth exploring further.
- Facilitates more straightforward communication of results.
- Often relates closely to p-values, guiding the interpretation of research findings.
- Statistical significance alone doesnāt ensure practical significance.
- Contextual interpretation is vital to evaluate the relevance of results.
In sum, advanced statistical techniques enrich oneās ability to analyze data effectively, guiding decision-making. Investors and analysts who grasp these concepts can better navigate the complex world of numbers, leading to outcomes that are not just statistically sound but also practically relevant.
Critical Thinking and Statistics
Critical thinking serves as the backbone of statistics education, playing a pivotal role in shaping how one approaches data interpretation and analysis. In a world flooded with information, the ability to sift through data critically can make all the difference. For any investorābe it a seasoned player or someone dipping their toes into the financial watersāutilizing critical thinking skills can enhance both understanding and decision-making.
Statistical results are often peppered with uncertainty, variability, and potential biases. Without the ability to critically evaluate these results, it becomes all too easy to draw incorrect conclusions. This is where the intersection of critical thinking and statistics truly mattersāby enabling students and professionals alike to scrutinize data rather than merely consume it.
Some key benefits of fostering critical thinking in statistics include:
- Enhanced Decision-Making: Better data evaluation leads to smarter investment strategies and outcomes.
- Improved Problem-Solving Skills: Engaging with data fosters a mindset bent on finding solutions rather than succumbing to confusion.
- Greater Awareness of Biases: Understanding how personal or external biases can influence data interpretation helps in mitigating their impact on decision-making.
Thus, to engage with statistics effectively, one must wield critical thinking like a seasoned toolmaker wields a chiselāsharp and precise.
Interpreting Statistical Results
Interpreting statistical results is not just a matter of looking at numbers; itās an art and a science rolled into one. Whether you're gazing at a simple mean or calculating the complexities of a regression model, the key lies in understanding context along with methodology. Just because a result looks appealing on the surface doesnāt mean it stands up to scrutiny when examined closely.
When evaluating statistical results, consider the following:
- Context Matters: Always ask, "What does this data mean in real-world terms?" The world is not black and white; numbers often tell a larger story.
- Understand the Methodology: The way data is collected, analyzed, and presented can drastically affect outcomes. Familiarity with sampling methods and analysis techniques is crucial.
- Check for Correlation vs. Causation: Just because two variables move together doesn't mean one causes the other. A classic saying is, "Correlation does not imply causation."
- Consider the Source: Who collected the data, and what biases may come into play? Knowing the background can shed light on potential issues hidden within results.
"Statistics is like a bikini. What is revealed is interesting; what is concealed is crucial."
Avoiding Common Statistical Fallacies
Understanding how to dodge common statistical fallacies is paramount for anyone venturing into the realm of statistics. Just as a pilot reviews their flight manual carefully, so too should an investor familiarize themselves with the pitfalls of statistical reasoning.
Here are some prevalent fallacies to watch out for:
- The Gamblerās Fallacy: Just because red has come up five times in a row doesnāt mean black is next. Each spin of the wheel is an independent event.
- Cherry-Picking Data: Selecting only certain data points that support a conclusion while neglecting others is misleading. A complete dataset offers a clearer picture.
- Ignoring Relevant Variables: Often termed as the "omitted variable bias," failing to consider other influencing factors can skew the results significantly.
- Overgeneralization: Drawing broad conclusions from a small sample is risky business. Always ensure that your sample size is representative and adequate.
By being aware of these fallacies, individuals can navigate through the murky waters of data interpretation with clarity and confidence, emerging not just informed but empowered.
Closure: The Impact of Statistics on Decision Making
Statistics plays a pivotal role in shaping decision-making processes across various domains, from business to personal finance. Understanding these influences carves pathways to informed choices, contributing significantly to both individual and societal progress. In this article, we have traversed through a spectrum of statistical concepts and techniques, underscoring their implications for everyday decisions. Notably, the ability to draw meaning from numbers and data enables stakeholders to navigate complexities with greater assurance.
The impact of statistics can be categorized into several critical areas:
- Informed Investment Choices: Investors equipped with statistical knowledge can dissect market trends and assess risks more adeptly, potentially increasing returns on investments.
- Data-Driven Business Strategies: Companies leveraging statistical methods can craft strategies rooted in performance metrics, ensuring more effective operations.
- Public Policy Development: Government agencies rely on statistical analysis to create policies that respond to community needs, fostering societal welfare.
Moreover, considering the growing reliance on data in the digital age, it becomes evident that a strong foundation in statistics is not just beneficial but essential. The vast array of data generated demands individuals who can comprehend and act upon it wisely.
"Statistics is the art of never lying, but of knowing how to mislead." ā Paul F. Lazarsfeld
This highlights the dual nature of statistics; while it offers clarity, it also carries the weight of responsibility. Understanding the potential for misinterpretation is vital for ethical decision-making.
The Future of Statistics Education
As we look ahead, the landscape of statistics education is poised for transformation. Educational institutions must embrace innovative teaching methods that resonate with students. This involves integrating technology and hands-on experiences within the curriculum. For example, using software like R or Python can provide students with practical skills directly applicable in the job market.
Additionally, interdisciplinary approaches can enrich statistics education by connecting data analysis with fields such as environmental science, sociology, and economics. This broader outlook encourages learners to appreciate the multifaceted uses of statistics in daily life. The inclusion of real-world case studies can further bridge the gap between theoretical principles and practical application, instilling a robust understanding of statistical relevance in various contexts.
Embracing Statistical Thinking in Everyday Life
Statistical thinking is not confined within the walls of academia; it is a vital skill applicable in everyday life. From making informed grocery purchases to critically evaluating news reports, the principles of statistics allow individuals to sift through the noise.
Consider how a simple understanding of probability could help in assessing risks when embarking on a travel adventure. Would you opt for that budget airline or a trusted one based on prior statistical performance?
Here are a few practical steps to help integrate statistical thinking:
- Question the Source: Dive deeper into the origin of the data presented; is it credible?
- Analyze Samples: When presented with aggregated data, always consider the sample size and selection methods. Small or biased samples can lead to erroneous conclusions.
- Utilize Basic Tools: Excel or statistical calculators can simplify data analysis, making the intricacies of statistics more manageable for everyday decisions.
By equipping oneself with statistical acumen, one can better navigate the complexities of life, ensuring choices made are sound and rational.